How to Think About AI: 4 Things Artificial Intelligence Experts Want You To Know
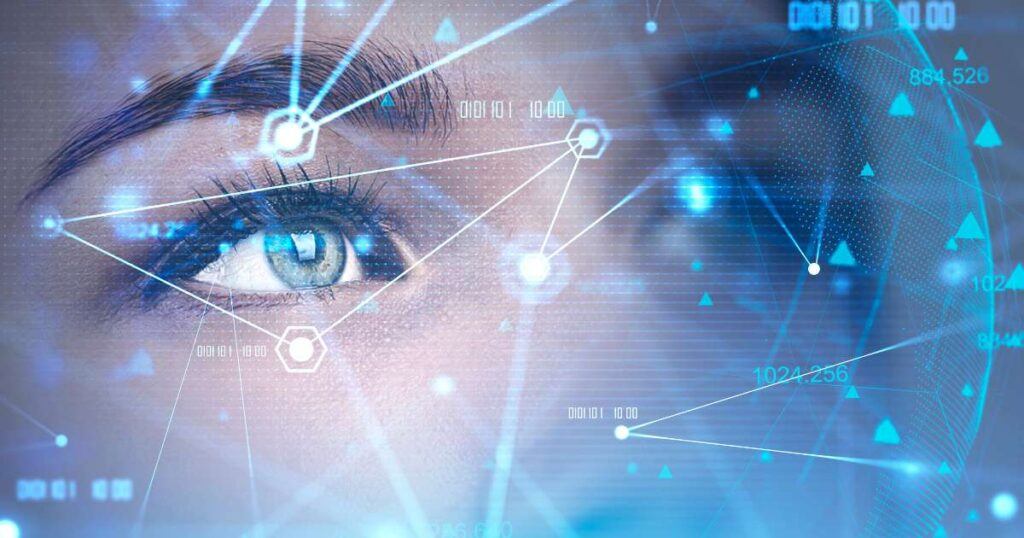
The most recent lurch forward in AI has introduced many people to the field. Each person coming to the field now has decades of understanding to digest, bringing their own spin for how they think about AI. They also have a huge sense of urgency when approaching AI in a business context. Given the rate of companies adopting artificial intelligence, this urgency is well-founded.
If you’ve just started to learn about artificial intelligence, welcome to the fold. Here are four things to keep in mind when thinking about artificial intelligence.
1. AI is a Spectrum
When people think about artificial intelligence for the first time, they tend to reach for sci-fi. Data, Bishop, and C-3PO are all classic examples you may think of. These are all examples of general intelligence. Objects that mimic the thinking of a human. They also exist on one extreme of the spectrum.
On the other extreme, we have the humble thermostat. A thermostat also exhibits common traits of an AI:
- It can observe its environment (the current temperature)
- It can plan for a desired outcome (the desired temperature)
- It can execute that plan (drive the heating and AC)
While the thermostat is a comically simple example of an intelligent autonomous agent, it’s also an illustrative one. If we only recognize artificial intelligence when it has near-human intellect, we risk missing simple but profitable opportunities.
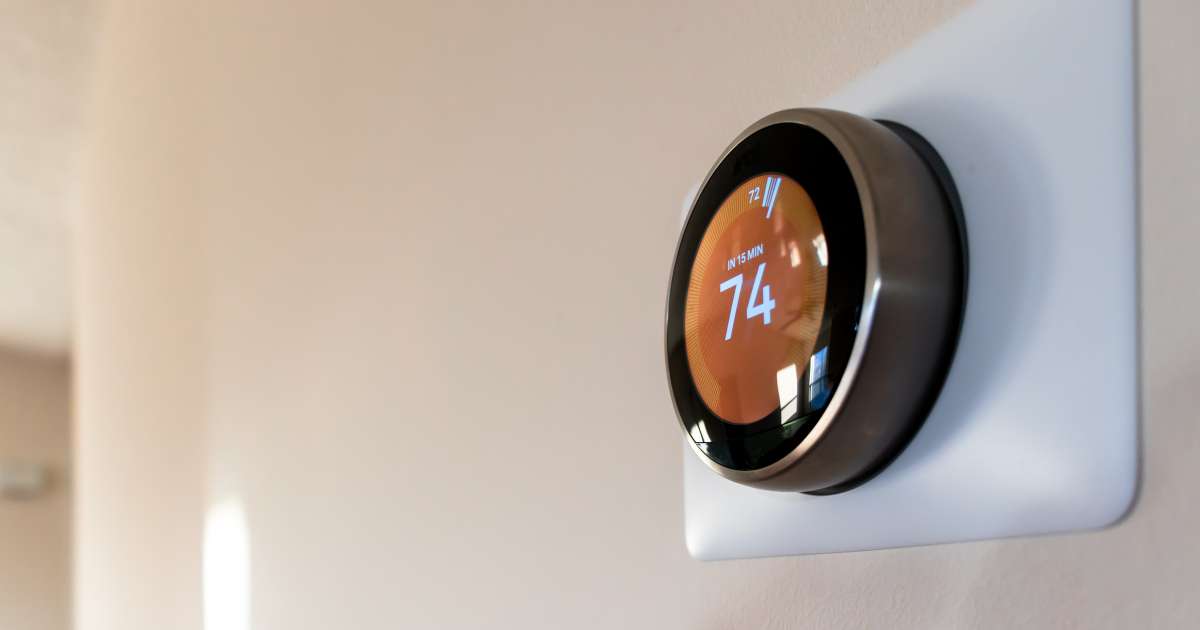
2. AI isn’t a Monolith
Given the current excitement, conflating artificial intelligence with large language models is tempting. However, LLMs are simply one type of AI. Artificial Intelligence is a huge field with over 70 years of results. These subdisciplines each address a unique set of problems. Each area has various techniques to handle any setting and constraints.
If we over-focus on the most recent advances, we risk missing out on chances to apply reliable, tested techniques from yesteryear.
3. Automation Isn’t All Or Nothing
When we think about artificial intelligence, it’s tempting to imagine something whirling away in the corner. Something operating uninterrupted and unassisted, as the days and years tick by. Certainly there are systems that operate in this way, for example the tools used in lights-out manufacturing. However, much like how the sophistication of AI is a spectrum, so too is the automation.
In AI, automation comes in three main varieties. Full automation, where the system runs without human intervention. In partial automation, artificial intelligence handles the easy cases and leaves the most difficult instances for humans. Finally, decision support systems include no automation. Instead, they assist humans in making complicated decisions.
Each flavor of automated AI deployment seeks to achieve the same goals:
- Increase the amount of work done
- Reduce the cost of doing work
- Reduce the failure rate of the work
The opportunities to drive these metrics exist in all sorts of work. If we restrict ourselves to only the fully-automatable case, we risk missing out.
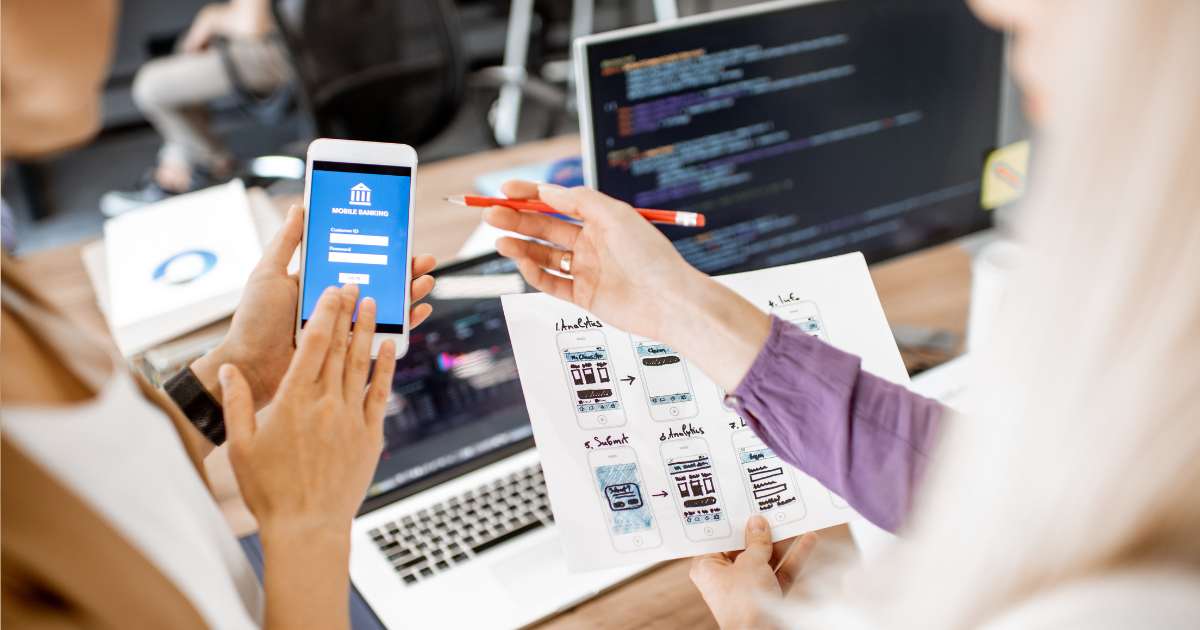
4. You Build AI Software Like All Software
While artificial intelligence is different than many other types of software, it is, at the end of the day, software. Many of the best practices the software industry has developed over the years apply here. And, we should use them.
When building AI systems, it’s still a good idea to have cross-functional teams. There are a number of ways to solve any business problem. Keeping AI folks at the table in product and engineering meetings allows you to select the best approach fit to the task, and course correct as needed.
AI lends itself to the same agile mentality as other software development. It’s best if the components of a system can continually deliver value. You should divide artificial intelligence work into bite-sized pieces and demo progress regularly.
Bringing It All Together
Artificial Intelligence has been around for decades and will be around for decades more. Recent advances in large language models have brought renewed interest in AI. With that renewed interest comes a surge of folks just starting to grapple with AI. If that’s you, remember:
- AI is a spectrum from narrowly to generally intelligent
- Automation is a spectrum as well
- AI is a collection of many techniques, not a monolith
- AI is just software, and current best practices still apply
If you’re interested in learning more about Artificial Intelligence, please reach out! We’re always eager to talk about how AI can be used to responsibly solve novel problems.
Unlock the Power of AI Engineering
See how we apply custom AI software solutions, from optimizing manufacturing materials to analyzing and predicting equipment maintenance schedules.
You Might Also Like